
Generative AI chatbots are revolutionizing customer service by delivering fast, personalized and automated interactions. One of the most important elements enabling the effectiveness of chatbots is memory management, which allows chatbots to maintain context and provide consistent, personalized responses. This blog explores the importance of memory management, types of memory and how LeverBot is improving it with innovative solutions.
Importance of Memory in Chatbots
Effective memory management plays a crucial role in the success of chatbots. With proper memory usage, chatbots can:
- Track Context: Remembering users’ previous messages allows chatbots to provide accurate and relevant responses.
- Offer Personalization: By remembering user preferences, chatbots can offer tailored recommendations.
- Solve Problems Quickly: Using past information, chatbots can prevent repetitive data input and save time.
- Strengthen Customer Relationships: Continuous interaction with users helps increase customer loyalty.
Types of Memory
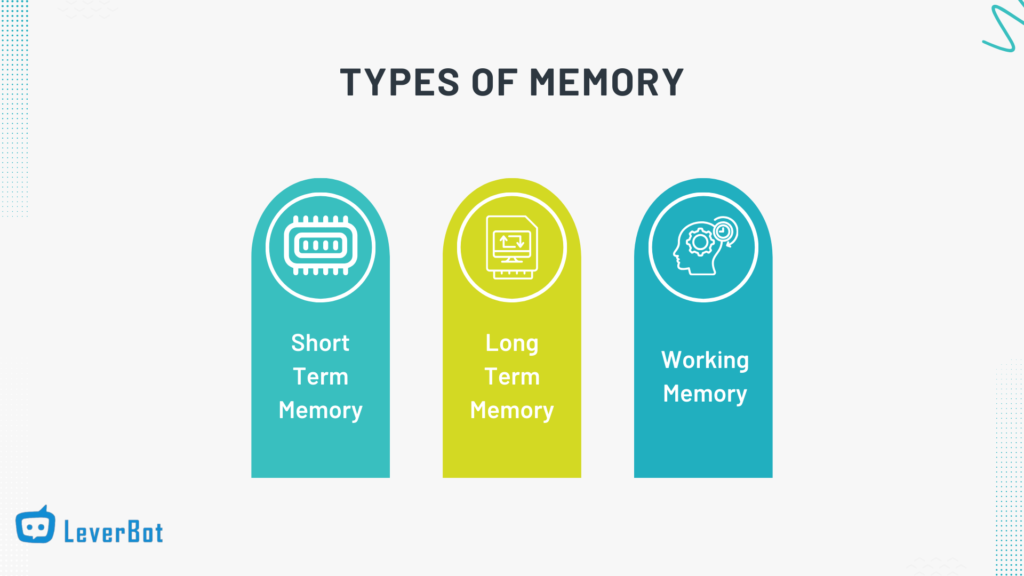
Short-Term Memory (STM)
Short-term memory holds information during real-time interactions with the user. This memory type is used temporarily from the beginning to the end of the conversation. For example, the response to a user’s question is stored during the session, but this information is lost once the session ends. This allows the chatbot to quickly resolve repeated questions within the same session.Long-Term Memory (LTM)
Long-term memory allows the chatbot to store information learned from past interactions. User preferences, habits, or previously resolved issues are kept in long-term memory. This enables the chatbot to provide more personalized service. For instance, if a user has previously purchased a particular product, the chatbot can remember this and use the information in future interactions.Working Memory
Working memory contains information that is processed and used temporarily during tasks. This type of memory is used to store information necessary for completing tasks during a conversation. Working memory is crucial for responding to complex questions or handling multi-step processes. For example, if a user is continuously entering information during a reservation process, the chatbot temporarily stores this data.
LeverBot leverages these memory types together to enhance chatbot efficiency and improve user experience.
LeverBot Approach to Memory Management
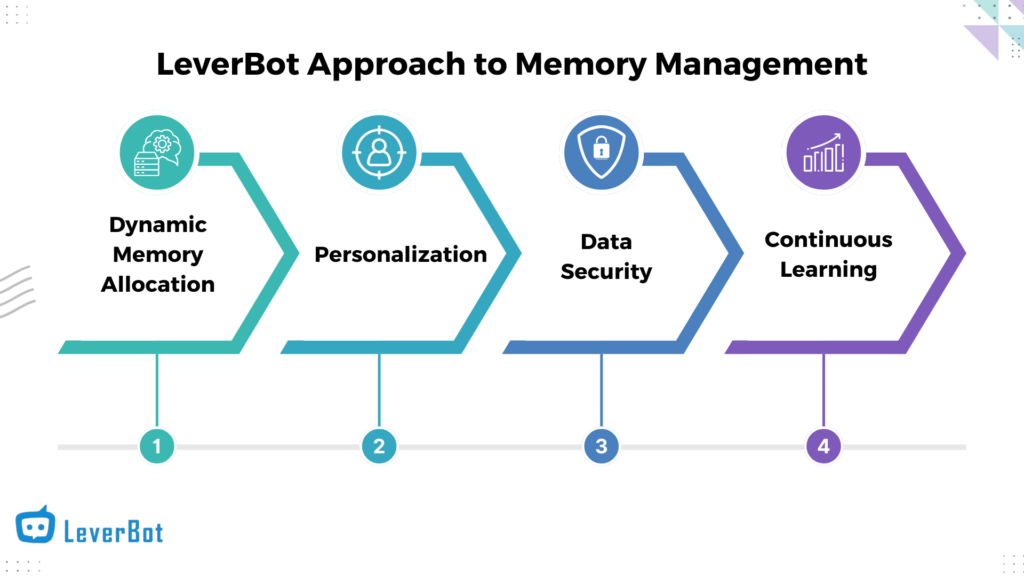
LeverBot offers advanced memory management strategies that enhance chatbot performance:
1. Dynamic Memory Allocation
Chatbots allocate resources based on conversation complexity, ensuring efficient memory use.
2. Personalization
By leveraging long-term memory, LeverBot delivers personalized, user-specific interactions, enhancing customer satisfaction.
3. Data Security
LeverBot prioritizes secure data handling, safeguarding user information with encryption and access controls.
4. Continuous Learning
LeverBot’s chatbots learn from interactions, improving their ability to handle context and provide accurate responses over time.
Conclusion
Memory management is essential for generative AI chatbots to deliver context-aware and personalized experiences. LeverBot addresses challenges like data privacy, context tracking, and memory constraints through innovative solutions. By prioritizing security, personalization, and continuous improvement, LeverBot empowers businesses to elevate customer service with AI-driven chatbots.
Visit LeverBot to explore how these solutions can transform your customer service strategy.